Reaching Higher Accuracy in Long-Term River Flow Forecasting
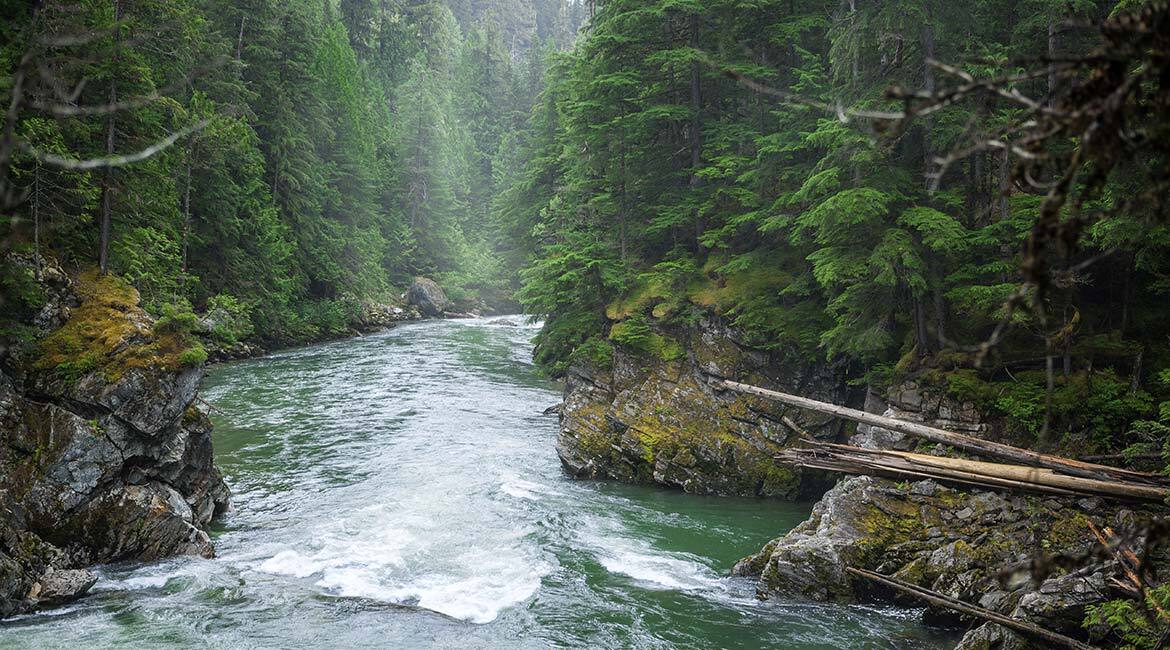
Purchased on Istockphoto.com. Copyright.
River flow forecasting is a critical task in water resources management for municipalities and hydropower operations. A well-established method called ESP (Ensemble Streamflow Prediction) is currently used to predict long-term river flow forecasting. It uses historical climatological data as an estimator of possible future weather patterns to predict river flows. The probabilistic nature of ESP means that the outputs are highly uncertain. Many studies have investigated the uncertainty and decreased it in numerous ways, for example by removing less probable scenarios in light of the atmospheric system at the forecast issue date. This study proposes an algorithm to quantify the potential of historical data in predicting river flows. The Genetic Algorithm (GA) was used to optimize which scenarios to use given a number of potential historical weather scenarios. Forecasters can use this method to evaluate the potential of using historical data as their forecasting method, which will help develop new processing avenues to attain these optimal targets. Keywords: River flow forecasting; Ensemble Streamflow Prediction; Genetic Algorithm; Hydropower; Flood forecasting
Hydrology is the study of water cycle processes [1]. In other words, it finds answers to questions like “how much water will flow through River X at City Y in the next month?” This article is about hydrological forecasting, specifically streamflow prediction. Hydrological forecasting is a unique, crucial tool for modern society. It affects the safety of populations in different ways, such as saving lives by issuing flood warnings or predicting upcoming droughts. And it is not always about extremes: Agriculture (food security) and Hydropower (energy security) are also two important sectors that benefit from streamflow forecasting.
Improving Forecasts with Historical Data
A widely used method to predict streamflow is called ESP (Ensemble Streamflow Prediction). ESP is a probabilistic method [2] [3] [4] since it does not provide a unique estimate of the level of upcoming water. Instead, ESP projects a probabilistic answer, using historical events (ensemble members) that are possible realizations of upcoming flows. The probabilistic nature of ESP renders uncertainty unavoidable, but methods exist to reduce uncertainty as much as possible and increase its accuracy. Many studies have been performed to improve ESP forecasts. These methods remove less probable scenarios to issue more successful forecasts. For example, the historical weather scenarios used in ESP could be dependent on the hydrological state at the forecast issue time—if it has been raining for the past few days, the hydrological conditions will be different than in dry conditions—or incorporating large-scale climate indices like El-Nino [5] [6] [7], which might modify the atmospheric state and increase the odds of having a rainy or dry season for example. This study presents a method that evaluates the potential of historical data in predicting future streamflows by optimizing historical weather scenario selection using the Genetic Algorithm (GA).
This study proposes a way to optimize the use of historical data by finding the best possible strategy a posteriori. For example, to estimate the volume of water that will enter the Lac-Saint-Jean hydropower reservoir in the next 90 days, we can select from 65 years of historical weather records and, considering the forecast date climate conditions, we can choose the 30 most likely scenarios to perform ESP. This forecast is Skill A. On the other hand, if we wait for 90 days and then repeat the process once more but taking into account what the actual volume was, we can optimize our selection with 30 members from the historical records using GA. This optimized forecast is Skill B. There is no doubt that B is better than A, since it was optimized using information that was unavailable during the forecast date. In this study, we quantified this improvement of B over A and called it the “unrealized potential of historical data” or simply delta (∆). Delta (∆) is shown in figure 1. Regarding figure 1, it is important to know that the lower the CRPS, the better. Interested readers can learn more about CRPS, a measure of forecast skill, in [8]. Repeating the process of finding the best set of m historical data scenarios to minimize the corresponding CRPS—maximizing forecast performance—was done by using GA for all values of m.

Figure 1 — Illustration of forecast improvement potential in the form of CRPS—a forecast skill that calculates the difference between target streamflow and forecasts. Lower is better.
∆CRPS is the unrealized potential of historical data; X-axis, the forecast skill; Y-axis, the number of historical weather scenarios in the ensemble.
For each watershed, the GA optimizer determines which years of the historical data to select to obtain the best possible forecast skill. Figure 2 and 3 show results for a complete range of historical scenarios in Québec (figure 2) and British Columbia (figure 3).

Figure 2 – Showing theoretical potential of the ESP method for different sizes of ensembles; catchment located in Quebec.
X-axis: forecast skill; Y-axis: number of historical weather scenarios in the ensemble

Figure 3 – Showing theoretical potential of the ESP method for different sizes of ensembles; catchment located in British Columbia.
X-axis: forecast skill; Y-axis: number of historical weather scenarios in the ensemble
The average improvement in forecast skill obtained by removing the most unlikely scenario is larger for the QC catchment. This means there is more unrealized potential in forecasting streamflow in QC catchment than in BC. This was expected because BC’s climate is less variable than in Québec.
Conclusion
Improving hydrological forecasts translates into saving human lives, ensuring energy security and reducing impacts on the environment. This study presented a novel way to estimate the maximum value contained in historical weather records to improve long-term streamflow forecasts. The method developed in this study lets forecasters know how much more potential is available in their datasets and will allow them to improve their methods until they can attain this optimal target. It will also help fuel future research in this field as there is now a finality to possible improvement, which was not the case before.