AI Controlled Drone for Wireless Service Provisioning

Purchased on Istockphoto.com. Copyright.
In this article, we investigate wireless service provisioning through a rotary-wing Unmanned Aerial Vehicle (UAV), which can serve as an aerial base station (BS) to communicate with multiple ground terminals (GTs) in demand boost areas. Our objective is to optimize the UAV control to maximize UAV energy efficiency, where both aerodynamic energy and communication energy are considered while ensuring communication requirements for each GT and a backhaul link between the UAV and the terrestrial BS. UAV and GT mobility lead to time-varying channel conditions that make the environment dynamic. We formulated a nonconvex optimization to control the UAV considering the practical angle-dependent Rician fading channels between the UAV and GTs, and between the UAV and the terrestrial BS. Traditional optimization approaches cannot handle dynamic environments and high complexity of the problem in real time. We propose to use a deep reinforcement learning-based approach, namely Trust Region Policy Optimization (TRPO), to solve the formulated nonconvex problem of UAV control with a continuous action space that takes into account the environment in real time, including time-varying UAV-ground channel conditions, available UAV onboard energy, and GT communication requirements.
Providing Communication Services with Drones
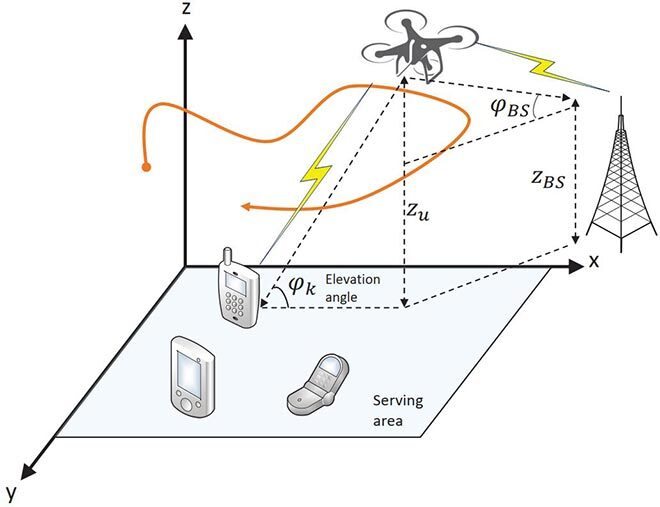
Figure 1: Illustration of our system model.
The development of unmanned aerial vehicle (UAVs) technology is emerging to enable 5G systems to provide reliable and ubiquitous connectivity to mobile users. In particular, UAVs equipped with onboard wireless transceivers can fly over a target area and provide communication services especially in the areas where deploying terrestrial base stations (BSs) is difficult or communication infrastructure are disaster-stricken. Thanks to their high manoeuvrability, UAVs can adjust their aerial position according to real-time locations of ground terminals (GTs) for energy efficiency and improved communication performance. Moreover, by flying over GTs at a given altitude, UAV-enabled communications can achieve better channel quality since communication links with GTs are mainly controlled by line-of-sight (LoS) links. For example, a UAV flying at an altitude of 120 m in a rural environment can provide air-to-ground links with a LoS probability exceeding 95%. Therefore, UAV-enabled wireless communication becomes a promising cost-effective paradigm for 5G systems by enabling on-demand operations and facilitating fast and flexible deployment of communication infrastructure.
Along with these advantages, UAV-enabled wireless communication systems face many challenges. In particular, operating the UAV, which fundamentally depends on limited onboard energy according to aircraft and onboard battery size. Therefore, it is necessary to define an effective and efficient mechanism to use this limited energy in order to enhance communication performance and prolong UAV endurance. Compared to conventional terrestrial BSs, UAVs incur additional propulsion energy consumption to remain airborne and support their movements. Moreover, UAV and GT mobility result in time-varying channel conditions which make the environment dynamic. Therefore, designing an energy-efficient UAV-enabled wireless communication system becomes more difficult and significantly different from conventional terrestrial communication systems.
To overcome the limited onboard energy challenges, we propose to leverage emerging deep reinforcement learning (DRL), which has been shown to provide superior performance in handling a time-varying environment with sophisticated state space. DRL uses powerful deep neural networks (DNNs) to produce a stationary optimal control policy without requiring complete knowledge of dynamic environmental statistics.
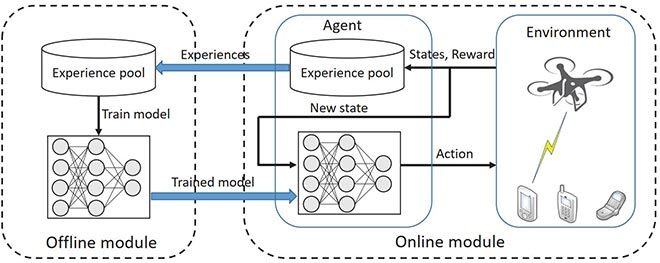
Figure 2: Proposed two-module TRPO-based framework
for practical implementation.
Deep Reinforcement Learning Approach
The problem of UAV control can be formulated as a Markov Decision Process (MDP), as follows:
- System states: The network state in time slot t can be characterized by the channel power gain between the UAV and GTs, the available onboard energy of the UAV, and the remaining data requirement of the GTs at time t.
- Actions: The action of the UAV at time t is the horizontal and vertical velocities.
- Reward: In RL, the reward function should be related to the objective function. Consequently, we designed a reward as the combination of ground terminal achievable data rate and UAV energy consumption.
Our simulation results are illustrated in Figure 3.

(a) Average reward (b) Energy consumption (c) Total achievable data rate (d) Energy efficiency
Figure 3: Performance of the proposed DRL scheme.
Conclusion
In this article, we propose a deep reinforcement learning-based approach for UAV control with the objective of achieving energy consumption minimization. Numerical results reveal that the TRPO-based algorithm can improve performance compared to the DDPG-based algorithm in a highly dynamic environment, which is the case in this paper. Moreover, both TRPO-based and DDPG-based algorithms outperformed the baseline ‘Q-learning’ and heuristic algorithm in terms of energy efficiency.
Acknowledgments
The authors thank Mitacs, Ciena, and ENCQOR for funding this research under the grant IT13947.
Please find the full article under the following reference [1].