Adopting a Healthy Lifestyle with the help of Artificial Intelligence
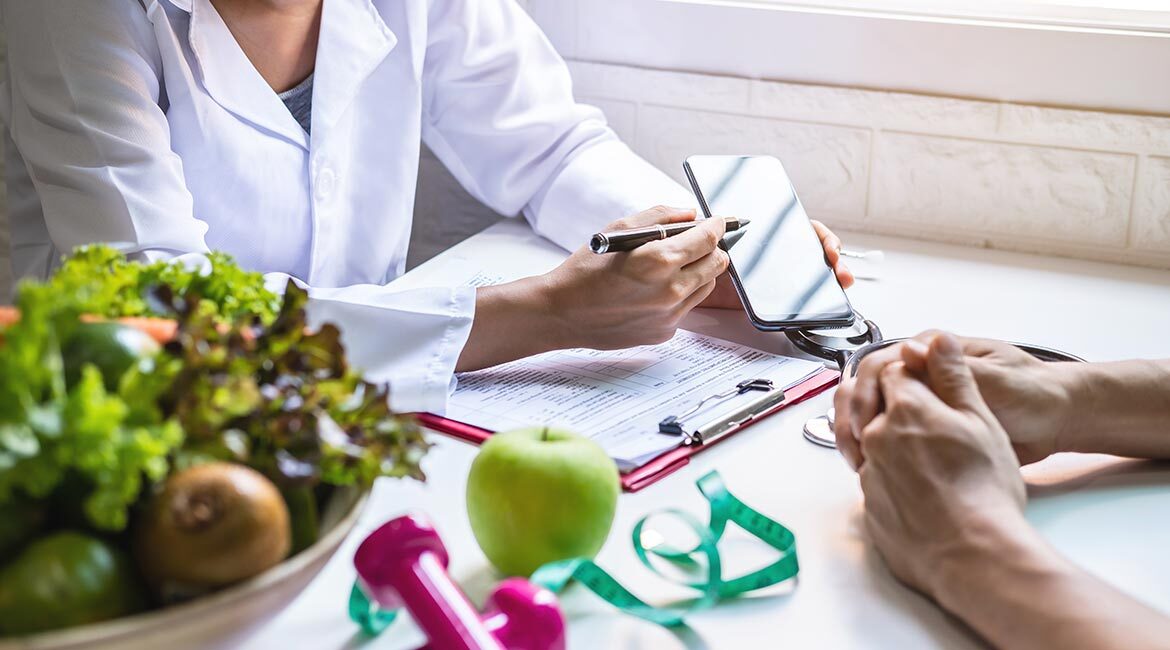
Purchased from Istockphoto.com. Copyright.
Non-communicable diseases—heart conditions, cancer, diabetes and obesity—are responsible for 70% of deaths worldwide (88% in Canada), even in the context of pandemics. Despite constant increases in health care budgets, these figures continue to rise because these types of diseases are mainly caused by poor lifestyle habits such as sedentary lifestyle, poor diet, and smoking. Even though Canadians know that it is important to be physically active, to eat fruits and vegetables, and to quit smoking, many fail to put this into practice. This gap between attitude and behaviour demonstrates the difficulty in making sustainable lifestyle changes.
Ambivalence – The Barrier to a Healthier Lifestyle
Ambivalence is defined as the gap between the desire to change behaviours and the perceived barriers to doing so, e.g. “I know exercising would be good for me, but I can’t find the time to do it.” Specific means to address ambivalence to change, through motivational communication, have proved very effective in inducing long-term, sustainable change. However, this form of intervention is traditionally delivered face-to-face, which has limited its access to a significant portion of the population.
Simon Bacon, Professor of Behavioural Medicine at Concordia University and Researcher at the CIUSSS-du-Nord-de-l’Île-de-Montréal Research Centre, is among the developers of a health application called ACCELERATION. The goal of this online health platform is to help patients follow a wellness plan and adopt healthier habits, and it operates without human intervention. It uses motivational communication to increase intrinsic motivation and reduce ambivalence about adopting healthy habits. The first version showed encouraging results, though they varied greatly from one person to another. Joining the project to increase ACCELERATION’s intervention capacity is Éric Granger, Professor of Systems Engineering at ÉTS and a member of the Laboratory for Imagery, Vision and Artificial Intelligence (LIVIA). His goal is to use artificial intelligence to interpret non-verbal language, such as ambivalence, including distress or demotivation among users of online e-health services.
Undeniably, recent advances in machine learning and computing power now make it possible to develop technologies capable of detecting ambivalence in real time. As part of this project, interpreting ambivalence and other relevant emotional expressions will be based on the way participants respond to a series of questions put to them by an avatar. The conversation will provide basic data to train the artificial intelligence system.
Automatic Expression Detection
In face-to-face interviews, a participant’s body language, such as facial expressions, shoulder shrug, lack of participation, or hesitant tone of voice, will alert the questioner to signs of ambivalence. When this happens in the context of an e-health service (without human intervention), the process must be automatically adjusted to counter this ambivalence. This is the basis of integrating ambivalence detection in ACCELERATION.
Though some vocal and facial expression recognition systems already exist, they are not very robust, nor very accurate, and are limited to specific and basic emotions: joy, sadness, anger, etc. Ambivalence is a more subtle emotion that requires a set of more representative data in order to create a more refined recognition model. Using specialized deep learning models will make it possible to accurately recognize a specific emotional state from a combination of facial and vocal expressions, as well as posture. This ambitious project has several challenges to overcome.
Building Models without Annotations
Fundamentally, there are no relevant data about expressions of ambivalence in public databases. What’s more, the manual collection and annotation of expressions that Professor Bacon and his team need to perform are both painstaking and expensive processes. The deep learning models are therefore trained on weakly annotated data or refined with unannotated data (no annotation).
Fusion of Different Data Sources
Facial expressions, verbal expressions, and posture all provide important and complementary cues to a person’s state of mind. The fusion of time/space multimodal data (visual and audio) extracted from videos will have to be carried out to improve the accuracy and robustness of automatic expression detection.
Model Customization of Individual Expressions and Environment
Finally, the deep learning models need to be customized to include differences in individual expressions based on gender, ethnicity, race, age, culture, etc. They must be tailored to capture conditions like types of sensors, devices used, and environment in which the images and sounds are collected.
A High-Level Potential Solution
The main objective of this double research chair’s project is to adapt e-health interventions in response to ambivalence arising during a process of behaviour change. Seeing as ambivalence has repercussions on other health issues like therapeutic compliance or following health measures, the developed models could easily be adopted by other behaviour change applications.
In addition to extending patients’ lives and improving their quality of life through successful lifestyle changes, this project also has the potential to save significant amounts of public money in health care costs. The students to be trained by this project will be well positioned to fuel future growth in this field.